Common Mistakes in Data Science Training and How to Avoid Them
- Saniya Sharma
- Jan 17
- 3 min read
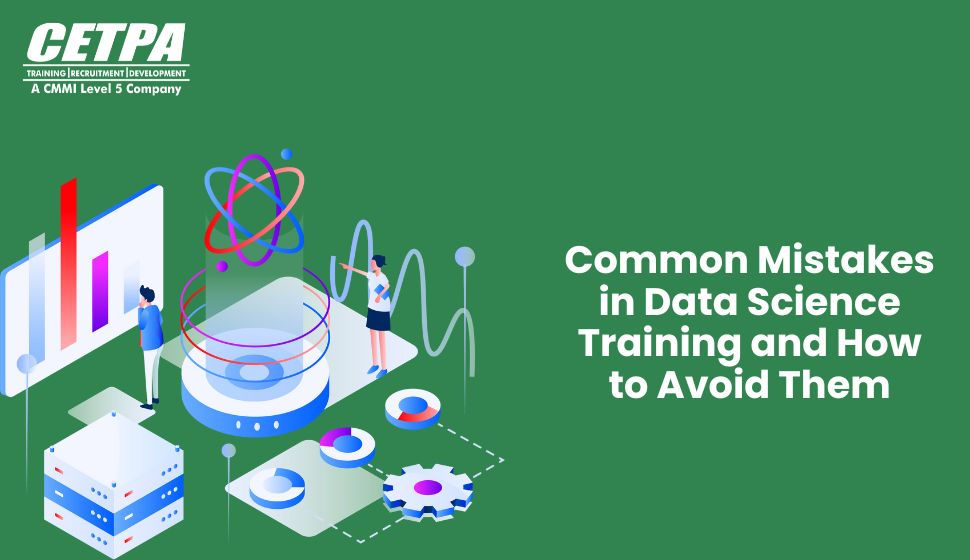
As a beginner in the field, you may be wondering what can go wrong with your data science model. Sometimes disorganized ways of handling data results in poor results or unexpected outcomes. Here is a list of some common errors that you as a data science specialist may commit. Go through each and avoid them in your professional journey.
Mistakes Causing Un-Expected Outcomes In Data Science
Having Disorganized Plans
Most of the time, whenever something fails, it happens due to not having a proper plan. What makes it even more challenging is when you are not able to divide the time effectively. Whenever professionals work with data science problems, the answer is to see the patterns and go for storytelling the way data is representing it. Data Science Training in Delhi enables the learners to learn about the professional world. These help beginners and experts to create and develop how they perform and fulfill job obligations.
To answer the questions in-depth, one has to be clear about what kind of methodology is to be followed. Herein, separately they are required to be more proficient with the kind of questions that are to be answered and how to answer them. Furthermore, they may also incorporate plans to understand the kinds of ordeals which helps make them reflect on the latest data management practices.
Failing to Keep Account of Bias in Data
In data science there is a popular saying, that the results can be as good as the data may get. Most of the time you cannot contain data inside the space that supports the features of your choice. Therefore, whenever you work on data, the problems are to be solved using the data stored with the characteristic storage advantages of the space.
Devote extra time toward identifying and locating the inherited biases that make up the structure of data. Doing so helps avoid ending up with intentionally skewed models. The biases of not being handled correctly fail to present an accurate picture of what is going around. Data Science Course enables the learners to create and develop the learned means with which they learn to perform better alternatives using the best resourceful awareness.
Not Optimizing Data Enough for Data Model
The models are required to be optimized for the data they are following. Furthermore, the models must follow through with the changes that are reflective of the data stored in vaults. Follow the changes in data over time. Herein reflect on the developments through an easy to accentuate services following and managing the needs requirements.
In machine learning, it follows optimizing values of the hyperparameters to follow the model in deploying data parameters. Every time, the models require accurate optimizations. The optimizations follow through with the requirements of parameters analysis for predictions and forecasting. Winter Online Training with Data Science helps learners get started in the field. It is the time for students to learn about the latest practices through practical applications.
Not Working on the Performance of Model
Mistakes are something all of us have made at a certain point of time in our careers. They are reflective of eth needs assessment following the nature of developments in creating sustained and steadfast results. Accuracy by any chance can’t be neglected. But so has the importance of having a high performing data model.
The accuracy of solutions depends on the quality of the algorithms. The accuracy of the solutions depends on the kind of algorithms you choose. Changing any of these will reflect on the accuracy of the results. Therefore, shift your focus on data and accuracy will follow. Data Science Online Course helps learners stay in touch with the digital learning means. Furthermore, it enables the participants to fine-tune their skills by taking assistance from online learning methods.
Conclusion
Many of the problems encountered by data scientists spring out of lack of knowledge and accurate skills development. Have a structural issue-resolving technique in the right set of space and further develop an understanding of dealing with data science problems.
Comments